面向化学品风险预测的计算毒理学软件比较研究
A Comparative Study on Computational Toxicology Software for Chemical Risk Prediction
-
摘要: 化学品风险评价是进行化学品环境管理和污染防治的前提,其关键在于化学品环境暴露和危害性数据的获取。仅采用实验测试获取相关数据的效率较低、成本高,难以满足数以万计的化学品风险评价的需求。以定量构效关系(QSAR)模型为代表的计算毒理学技术,可实现化学品环境暴露与危害性参数的高通量预测,是填补相关数据缺失的重要方法。近年来,计算毒理学领域发展了一批面向化学品环境暴露和危害性参数预测的软件工具,集成了数据和模型资源,在化学品管理活动中发挥了重要作用。本研究共搜集了国内外25款计算毒理学软件,通过文献资料调研和软件试用,从开发背景、预测终点、预测方法、功能与信息完整度和内嵌模型预测性能等方面对软件进行分析比较。分析表明,化学品管理法规支持、多方合作和数据共享,是计算毒理学软件开发的重要基础条件。现有计算毒理学软件在预测终点覆盖度、预测结果可靠性、软件实用性和可拓展性方面仍有提升空间。未来相关软件的开发需要结合深度学习等先进建模技术,增强软件的预测性能并扩大其应用范围,使之成为化学品风险综合评价和决策分析的实用工具。Abstract: Chemical risk assessment is important for chemical regulation and pollution prevention, the key step for risk assessment is to obtain the environmental exposure and hazard parameters of chemicals. Traditional experiments are time-consuming and costly to obtain these parameters, thus are impractical to assess the risks of a large number of chemicals. The quantitative structure-activity relationship (QSAR) model has drawn growing attention because it is a high-throughput computational toxicology method to predict such parameters. Recently, a set of computational toxicology software which integrate chemical parameters and computational model resources has been developed to predict environmental exposure and hazard parameters of chemicals, playing an important role in chemical management. This study collected 25 computational toxicology software and compared their development background, predicted endpoints, prediction methods, comprehensiveness of the information and functions, prediction accuracy through literature review and software trial. Results showed that the support of chemical management regulations, the multi-field cooperation and data sharing are important for development of computational toxicology software. Current software is generally limited in endpoint coverage, prediction reliability, software practicability and expansibility. To further develop such software for practical use in comprehensive chemical risk prediction and decision analysis, it is necessary to increase their predictive performance and expand their applicability via adopting advanced modeling techniques such as deep learning.
-
Key words:
- chemicals /
- risk assessment /
- computational toxicology /
- software
-
-
United Nations Environment Programme (UNEP). Global Chemicals Outlook Ⅱ:From legacies to innovative solutions:Implementing the 2030 agenda for sustainable development[R]. Geneva:UNEP, 2019 Landrigan P J, Fuller R, Acosta N J R, et al. The Lancet Commission on pollution and health[J]. Lancet, 2018, 391(10119):462-512 Wu S, Powers S, Zhu W, et al. Substantial contribution of extrinsic risk factors to cancer development[J]. Nature, 2016, 529(7584):43-47 王中钰,陈景文,乔显亮,等.面向化学品风险评价的计算(预测)毒理学[J].中国科学:化学, 2016, 46(2):222-240 Wang Z Y, Chen J W, Qiao X L, et al. Computational toxicology:Oriented for chemicals risk assessment[J]. Scientia Sinica Chimica, 2016, 46(2):222-240(in Chinese)
Wang Z Y, Walker G W, Muir D C G, et al. Toward a global understanding of chemical pollution:A first comprehensive analysis of national and regional chemical inventories[J]. Environmental Science&Technology, 2020, 54(5):2575-2584 Schmidt C W. TSCA 2.0:A new era in chemical risk management[J]. Environmental Health Perspectives, 2016, 124(10):A182-A186 Krimsky S. The unsteady state and inertia of chemical regulation under the US Toxic Substances Control Act[J]. PLoS Biology, 2017, 15(12):e2002404 Hartung T, Rovida C. Chemical regulators have overreached[J]. Nature, 2009, 460(7259):1080-1081 Kavlock R, Dix D. Computational toxicology as implemented by the US EPA:Providing high throughput decision support tools for screening and assessing chemical exposure, hazard and risk[J]. Journal of Toxicology and Environmental Health, Part B, 2010, 13(2-4):197-217 Egeghy P P, Sheldon L S, Isaacs K K, et al. Computational exposure science:An emerging discipline to support 21st-Century risk assessment[J]. Environmental Health Perspectives, 2016, 124(6):697-702 Collins F S, Gray G M, Bucher J R. Toxicology. Transforming environmental health protection[J]. Science, 2008, 319(5865):906-907 Pavan M, Worth A P. Publicly-accessible QSAR software tools developed by the Joint Research Centre[J]. SAR and QSAR in Environmental Research, 2008, 19(7-8):785-799 Jaworska J S, Comber M, Auer C, et al. Summary of a workshop on regulatory acceptance of (Q) SARs for human health and environmental endpoints[J]. Environmental Health Perspectives, 2003, 111(10):1358-1360 Organization for Economic Co-Operation and Development (OECD). Guidance document on the validation of (quantitative) structure-activity relationships[(Q) SARs] models[R]. Paris:OECD, 2007 European Commission, Joint Research Centre (JRC). JRC QSAR Model Database[DB]. Brussels:European Commission, 2020 Blaauboer B J. Biokinetic modeling and in vitro - in vivo extrapolations[J]. Journal of Toxicology and Environmental Health, Part B, 2010, 13(2-4):242-252 MacKay D. Finding fugacity feasible[J]. Environmental Science&Technology, 1979, 13(10):1218-1223 LaLone C A, Ankley G T, Belanger S E, et al. Advancing the adverse outcome pathway framework:An international horizon scanning approach[J]. Environmental Toxicology and Chemistry, 2017, 36(6):1411-1421 Ball N, Cronin M T, Shen J, et al. Toward good read-across practice (GRAP) guidance[J]. ALTEX, 2016, 33(2):149-166 Patlewicz G, Jeliazkova N, Gallegos Saliner A, et al. Toxmatch:A new software tool to aid in the development and evaluation of chemically similar groups[J]. SAR and QSAR in Environmental Research, 2008, 19(3-4):397-412 Dimitrov S D, Diderich R, Sobanski T, et al. QSAR toolbox:Workflow and major functionalities[J]. SAR and QSAR in Environmental Research, 2016, 27(3):203-219 陈景文,李雪花,于海瀛,等.面向毒害有机物生态风险评价的(Q) SAR技术:进展与展望[J].中国科学(B辑:化学), 2008, 38(6):461-474 Maunz A, Gütlein M, Rautenberg M, et al. Lazar:A modular predictive toxicology framework[J]. Frontiers in Pharmacology, 2013, 4:38 Contrera J F, Matthews E J, Benz R D. Predicting the carcinogenic potential of pharmaceuticals in rodents using molecular structural similarity and E-state indices[J]. Regulatory Toxicology and Pharmacology, 2003, 38(3):243-259 Luechtefeld T, Marsh D, Rowlands C, et al. Machine learning of toxicological big data enables read-across structure activity relationships (RASAR) outperforming animal test reproducibility[J]. Toxicological Sciences:An Official Journal of the Society of Toxicology, 2018, 165(1):198-212 Raies A B, Bajic V B. In silico toxicology:Computational methods for the prediction of chemical toxicity[J]. Wiley Interdisciplinary Reviews:Computational Molecular Science, 2016, 6(2):147-172 European Centre for Ecotoxicology and Toxicology of Chemicals (ECETOC).(Q) SARs:Evaluation of the commercially available software for human health and environmental endpoints with respect to chemical management applications[R]. Brussels:ECETOC, 2003 Lo Y C, Rensi S E, Torng W, et al. Machine learning in chemoinformatics and drug discovery[J]. Drug Discovery Today, 2018, 23(8):1538-1546 Vo A H, van Vleet T R, Gupta R R, et al. An overview of machine learning and big data for drug toxicity evaluation[J]. Chemical Research in Toxicology, 2020, 33(1):20-37 Rogers D, Hahn M. Extended-connectivity fingerprints[J]. Journal of Chemical Information and Modeling, 2010, 50(5):742-754 Mamy L, Patureau D, Barriuso E, et al. Prediction of the fate of organic compounds in the environment from their molecular properties:A review[J]. Critical Reviews in Environmental Science and Technology, 2015, 45(12):1277-1377 Netzeva T I, Worth A, Aldenberg T, et al. Current status of methods for defining the applicability domain of (quantitative) structure-activity relationships. The report and recommendations of ECVAM Workshop 52[J]. Alternatives to Laboratory Animals, 2005, 33(2):155-173 Mansouri K, Grulke C M, Judson R S, et al. OPERA models for predicting physicochemical properties and environmental fate endpoints[J]. Journal of Cheminformatics, 2018, 10(1):10 Todeschini R, Ballabio D, Consonni V, et al. Locally centred Mahalanobis distance:A new distance measure with salient features towards outlier detection[J]. Analytica Chimica Acta, 2013, 787:1-9 Maggiora G M. On outliers and activity cliffs:Why QSAR often disappoints[J]. Journal of Chemical Information and Modeling, 2006, 46(4):1535 Williams R V, Amberg A, Brigo A, et al. It's difficult, but important, to make negative predictions[J]. Regulatory Toxicology and Pharmacology, 2016, 76:79-86 Chakravarti S K, Saiakhov R D, Klopman G. Optimizing predictive performance of CASE Ultra expert system models using the applicability domains of individual toxicity alerts[J]. Journal of Chemical Information and Modeling, 2012, 52(10):2609-2618 Yordanova D, Schultz T W, Kuseva C, et al. Alert performance:A new functionality in the OECD QSAR Toolbox[J]. Computational Toxicology, 2019, 10:26-37 Tropsha A. Best practices for QSAR model development, validation, and exploitation[J]. Molecular Informatics, 2010, 29(6-7):476-488 Min S, Lee B, Yoon S. Deep learning in bioinformatics[J]. Briefings in Bioinformatics, 2017, 18(5):851-869 Linden L, Goss K U, Endo S. 3D-QSAR predictions for bovine serum albumin-water partition coefficients of organic anions using quantum mechanically based descriptors[J]. Environmental Science Processes&Impacts, 2017, 19(3):261-269 -
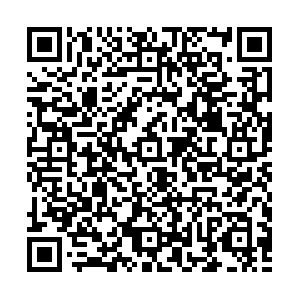
计量
- 文章访问数: 4768
- HTML全文浏览数: 4768
- PDF下载数: 234
- 施引文献: 0